ABOUT US
This course on Artificial Intelligence for Imaging is a unique opportunity to join a community of leading-edge practitioners in the field of Quantitative Medical Imaging. Whether you are a researcher in the field or are interested about fostering this type of research in your clinic, during this 4-days immersive course you will be able to attend lectures and workshops from world-class experts in Radiomics, Deep Learning and Synthetic Data. Clinicians will receive basic training in the methods of Quantitative Image Analysis and will be able to interactively design a clinical trial. Researchers will receive in-depth lectures about the state of the art and deeper training in commonly used algorithms. Researchers can also bring your own curated dataset for the hackathon (labelled, sorted by outcome, open source or fully anonymised, and cleared by ethics). If requested ahead of time, we will perform “data matching” for attendees to facilitate external cross validation. There will be ample opportunity to network with faculty members, other participants and companies.
Medical imaging has been the cornerstone for the management of patients for decades, particularly in oncology. Imaging data such as CT, MRI or PET are routinely acquired for every cancer patient in the process of diagnosis, treatment planning, image-guided interventions and response assessment. The use of image analysis in a quantitative way is now considered as one of the most promising techniques to support clinical decisions.
Quantitative Image Analysis looks at the phenotypic expression of genes, which results in particular imaging features or signatures able to characterize the imaged tissue and the underlying biology. By converting standard medical images into mineable data, the processes and methods of data science can be applied to them. Imaging features are distilled through machine learning into ‘signatures’ that function as quantitative imaging biomarkers. A major challenge for the community is the availability of data in compliance with existing and future privacy laws. Synthetic data and virtual clinical trial offer a solution to this issue and will also form a part of the methods explored in this course.
COURSE IN THE CONTEXT OF
The Marie Curie Network PREDICT, the NWO projects DuCAT and STRATEGY, the Interreg project EURADIOMICS
COURSE CONTENT
The course will be divided into lectures during the morning and hands on assignments in the afternoon. Parts of the course will be split into clinical and technical tracts, depending on your level of expertise. Participants of the hackathon are encouraged to come with their data and we will organize (if possible) matching data for validation from other participants on the course.
Our starting point is an overview of the history of Medical Imaging Artificial Intelligence we then discuss the success stories but also the pitfalls. Next, we will review the process from data acquisition, access to the DICOM objects, feature extraction, machine learning (including new developments with Deep Learning) analysis and validation.
The clinical tract will then learn more about the clinical implementation of quantitative imaging, from acquisition protocols to software solutions and finally the implementation of decision support systems.
The technical tract will focus on advances in synthetic data generation and harmonization techniques, new Deep Learning architectures, and current workflow solutions.
In the final part of the course, we will discuss the current challenges and directions of research in the field; in particular, the necessity of dealing with large annotated data sets, the FAIR principles and the distributed learning approach.
We offer you:
o Knowledge
o Skills and experience (e.g. in quantitative imaging analysis but also in outlining imaging related clinical trials)
o Network – connect to our AI4Imaging LinkedIn
o Documentation (papers, presentations, useful websites…)
o Possibility to spend time in the D-Lab in Maastricht to analyse own data
TARGET AUDIENCE
- clinicians in medical imaging (e.g. radiologists, oncologists, neurologists, cardiologists, ophthalmologists, dermatologists, ENT surgeons)
- medical physicists with an interest in research
- medical imaging researchers
- computer scientists with an interest in medical imaging
- academics researching quantitative imaging
LEARNING OBJECTIVES
Regarding Radiomics, Deep Learning and Synthetic Data (TECHNICAL TRACT) after this course you will be able to:
- Understand the fundamentals of big data analysis
- Understand the advantages and pitfalls of synthetic data generation
- Critically evaluate the literature and review published articles
- Understand how to implement a simple AI algorithm in order to answer a clinical question to augment a human decision
- Gain the tools to plan and evaluate an imaging-based clinical trial
- Gain basic understanding of regulation and privacy laws.
- Gain basic understanding of increasing the interpretability of AI models
ORGANISERS
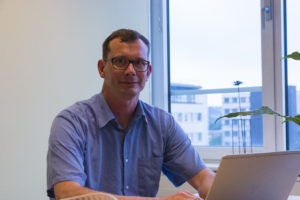
h.woodruff@maastrichtuniversity.nl

philippe.lambin@maastrichtuniversity.nl

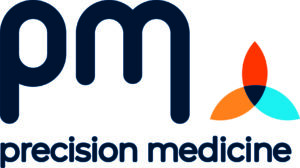
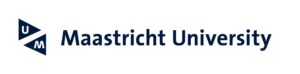
FACULTY 2024
- Henry Woodruff, Maastricht University, The Netherlands (Course Director)
- Anshu Ankolekar, Maastricht University, The Netherlands
- André Dekker, MAASTRO, The Netherlands
- Shahab Jolani, Maastricht University, The Netherlands
- Kaisar Kushibar, Universitat de Barcelona, Spain
- Philippe Lambin, Maastricht University, The Netherlands
- Andrew Maidment, University of Pennsylvania School of Medicine, USA
- Fanny Orlhac, LITO – Inserm/Institut Curie, France
- Zohaib Salahuddin, Maastricht University, The Netherlands
- Martijn Starmans, Erasmus MC, The Netherlands
- Wouter van Elmpt, MAASTRO & Maastricht University, The Netherlands
- Bram van Ginneken, Radboud UMC, The Netherlands
- Harini Veeraraghavan, Memorial Sloan Kettering Cancer Center, USA
- Alex Zwanenburg, NCT Dresden, Germany
VIDEOS
Eurocat
Decision Support Systems: of The D-Lab
Radiomics
Distributed learning
SPONSORSHIP
What are your benefits of sponsoring the course on AI4Imaging:
BRANDING
Invest in your brand equity by supporting our community
TALENT ATTRACTION
Connect with researchers, clinicians, engineers, analysts, data scientists at the forefront of AI, Imaging, deep learning, synthetic data and radiomics
THOUGHT LEADERSHIP
Demonstrate your company’s leadership and innovation chops in front of the brightest minds in the field
Come and tell our audience what your company has to offer them.
The earlier editions (2018, 2019, 2022 and 2023) were an enormous success with the maximum amount of participants.
The dedicated and tailored content of our course requires discussions and coding in a group setting and this functions best in physical attendance.
Consult our sponsorship prospectus 2024 or send your sponsorship request to Mieke at info@ai4imaging.org
SOCIAL PROGRAMME
All participants are invited to the course dinner on
Tuesday evening.
This event is for delegates and faculty only.
It is not possible to bring any accompanying persons. Pre-registration is compulsory.